Using ML to Deliver Medication Magnetically
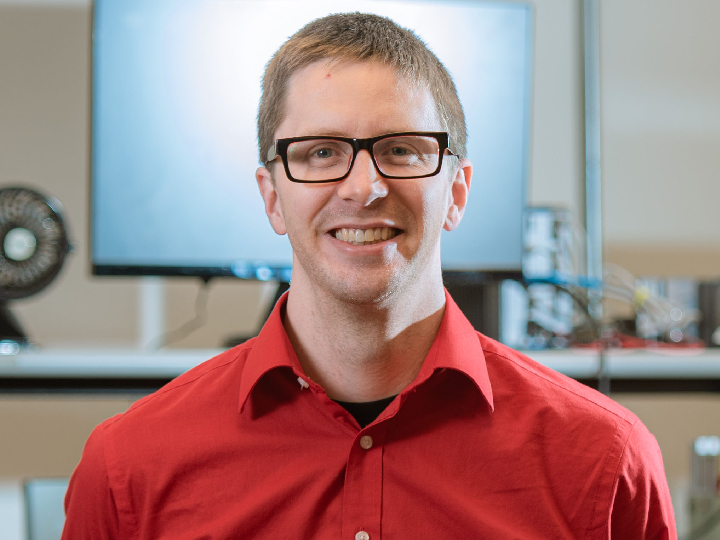
Today, cancer is a leading cause of death in the U.S., causing about one out of six deaths worldwide. Many patients undergo chemotherapy: a drug treatment that targets cancer cells, which spread and multiply quickly in the body. However, modern medicine often can’t tell the difference between cancer cells and other rapidly dividing ones. It’s why many types of chemotherapy have several side effects, such as alopecia, anemia, and peripheral neuropathy.
But what if we were able to target cancer cells directly? What if we could direct medicine to attack the tumor itself? Researchers like Aaron Becker, associate professor of electrical and computer engineering at the University of Houston, have joined forces to create more precise and controlled cancer treatments, converging methods of machine learning and targeted drug delivery. In his workshop for the HPE Data Science Institute, Becker highlighted work from his paper, “Gathering Physical Particles with a Global Magnetic Field Using Reinforcement Learning,” a joint collaboration between the Braunschweig University of Technology and the University of Houston.
In Becker’s experiments, a large swarm of micro-scale particles (“agents”) move through a maze that mimics the human vascular system in order to reach a target region representing a tumor. To deliver the particles to a desired location, Becker’s team used a time-varying magnetic field to gather the particles and reinforcement learning to train networks for optimal gathering.
The agents are trained using the Proximal Policy Optimization (PPO) algorithm in a modular Python environment, undergoing numerous simulations that mimic physical particles and real-world observations. Once trained, the agents are put through a series of hardware experiments in a lab-built magnetic manipulator. Two ablation studies— one focusing on simulations and another on a real-world experiment— tested the agents’ performance and robustness. Becker’s results found that, with this new approach, the gathering time for particles was significantly reduced from around half-an-hour to only tens of seconds.
Becker’s team has also utilized this method in other biomedical applications, navigating blood clot removals with magnetic swimmers. He shares his code on Github to encourage further extensions of this research and provide a valuable resource for the reinforcement learning community.